How AI will shape the future of risk management
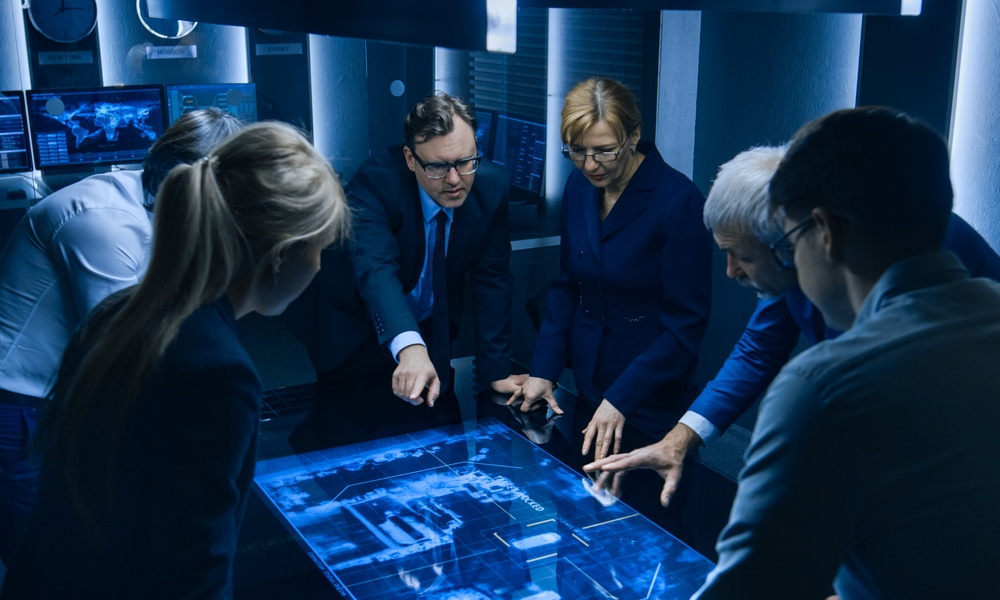
By Eric Boger, VP Risk Intelligence
It has become increasingly evident that the complexities and challenges that defined the risk landscape of 2023 will almost certainly persist throughout 2024 and beyond. Enterprises will continue to grapple with a relentless and intricate risk landscape; rather than facing isolated threats, they are confronted with a complex web of interconnected challenges. Some herald artificial intelligence in risk management as a remarkably potent solution, capable of addressing our most pressing challenges head-on. At Everbridge we maintain a more cautious stance, while diligently assessing the use of AI in the overall risk management landscape.
We are in an age of both polycrisis and permacrisis. Severe weather events have escalated in frequency, intensity, and unpredictability. Civil unrest and protests have surged. Geopolitical instability looms large.
The rise of deepfake technology, ransomware-for-hire schemes, and new avenues for manipulation and deception adds to the complexity of the situation. With deepfake videos and images, one can replicate images and voices of trusted individuals to circulate false information or drive unwise decision-making. Similarly, the proliferation of misinformation and disinformation further complicates the challenges we face.
As we navigate these intertwined and persistent challenges, it’s evident that while technology, particularly artificial intelligence for risk management, has played a pivotal role in worsening the problem, it may also hold the key to mitigating these complexities.
Advantages of AI
To be clear, the modern enterprise of threat detection would be very difficult without the assistance of AI and algorithmic processing. We find at least four (4) areas where automation and algorithmic processing is superior to human judgment and effort:
- Early indicator and weak signal detection
- Initial analysis and triage of potential event signals (at scale).
- Rapid geospatial orientation
- Text generation/Summarization
Early indicator and weak signal detection
AI-driven systems can detect subtle signatures or indicators of potential threats and risks within large datasets, helping organizations identify emerging patterns or anomalies that may signify potential threats. By continuously analyzing data and detecting these signatures, AI can provide early warnings and enable proactive responses to mitigate risks effectively.
Initial analysis and triage of potential event signals (at scale)
AI-powered systems are extremely proficient in conducting rapid analysis of large volumes of potential event signals. These systems leverage advanced algorithms to automatically triage incoming data, swiftly identifying and prioritizing potential threats or incidents based on predefined criteria. In addition to streamlining the initial analysis process, systems can offer threat categories and severity levels.
Rapid geospatial orientation
AI utilizes gazetteers and GIS systems to swiftly approximate and suggest the geographic location of potential risk events. This capability enables organizations to quickly orient themselves to the spatial context of emerging threats or incidents.
Text generation and summarization
AI systems can analyze the content and metadata of social media posts and news articles related to security events. The goal is to identify and extract information relevant to the nature of the threat. From here the better systems can analyze and synthesize the raw data into concise summaries.
However, the systems struggle with second-order thinking and the additional effort it takes to ensure intelligence of high accuracy, especially when tasked with precisely categorizing threats into different levels of urgency. Relying exclusively on AI reveals shortcomings, when it is charged with placing threats on the map with a strong degree of fidelity, or interpreting nuanced signals that might be easily misunderstood. AI systems, although advanced, are far from ideal – yielding intelligence of low to moderate confidence, ultimately leading to errors when high stakes decisions are made based on this quality of data alone.
Limitations of AI
We find at least four (4) areas where human judgment and effort outperforms automation and algorithmic processing (for now) in the realm of risk intelligence:
- Geospatial accuracy & precision
- Event classification & severity determination
- Temporal accuracy
- Source quality
Geospatial accuracy & precision
With structured metadata, AI systems can place events on a map well. However, most signals are nuanced and complex; in these cases, AI systems often defer to the geographic center of the nearest administrative boundary – city center or province center. Frequently, this is the best the system can produce. Human analysts leverage a variety of OSINT and GEO-orientation tools that require nuanced inputs. Human effort consequently allows for the placing of the event with precision.
Event classification & severity determination
Algorithms are good at “sensing” but often not “sense-making.” Making sense of a situation to determine the appropriate severity levels and characterization of the threat requires context. The intelligence professional will factor in the baseline narratives (current and historical) at the location and will evaluate proximate geographic features for exacerbating or alleviating factors.
This nuanced interpretation goes beyond the capabilities of AI, which typically scores and ranks threats based solely on programmed algorithms and lacks the human capacity to factor in the subtleties that can alter the gravity of a situation.
Temporal accuracy
Automated systems still get tripped up by current signals referring to prior events – sometimes years old. For current intelligence and security response, it is imperative to know if the event is cresting now or happened days ago.
Source quality
Geopolitical actors, adversaries, criminal groups, and even bored teenagers have an interest in pushing conflict and creating divisions. Misinformation and disinformation campaigns corrode the public discourse and deepen political or cultural rifts.
While some Al systems do a respectable job of identifying patterns that fit the signature of major disinformation campaigns, in more nuanced situations AI systems frequently get stumped by these efforts, and they don’t flag or suppress false information. Trained analysts fare better than machines at evaluating the reliability of a source and the credibility of the information delivered.
Challenges of AI in risk management
While AI systems have made significant advancements in categorizing risk events, they still face several challenges and shortcomings. One major limitation is the difficulty in accurately interpreting nuanced signals or contextual cues that may be crucial for understanding the severity or nature of a threat. AI systems may also struggle with detecting and mitigating emerging or previously unseen risks, as they rely on historical data for training and may not recognize novel patterns or trends. Plus, the inherent biases present in training data can result in biased or inaccurate risk assessments.
Additionally, data privacy may pose a significant concern in AI-driven risk management. As AI systems rely heavily on large amounts of data for training and decision-making, ensuring the privacy and security of this information becomes critical. Issues such as unauthorized access, data breaches, and misuse of personal information can undermine the integrity and reliability of AI-driven risk assessments. Integrating robust privacy measures into AI systems is essential to mitigate potential risks associated with data handling.
The Centaur Model for Risk Intelligence
The Centaur Model is a collaborative approach to intelligence analysis that combines the strengths of human analysts with the capabilities of AI algorithms. In Greek mythology, a centaur is a creature with the upper body of a human and the lower body of a horse, symbolizing the fusion of human intelligence and machine processing power.
In the Centaur Model, human analysts provide critical thinking, intuition, and contextual understanding, while AI algorithms excel at processing large volumes of data quickly and efficiently. Together, they form a symbiotic relationship where each complements the other’s strengths and mitigates weaknesses.
Everbridge’s Risk Intelligence Monitoring Center (RIMC) fully embraces the Centaur model.
In today’s intelligence landscape, there’s a dual pressure emerging from consumers: 1) fear of missing information and 2) concerns over the quality of data being compromised by poor processing or deliberate injections of mis- or dis- information.
Balancing these pressures is essential for accurate and actionable intelligence. AI alone can bring lots of data to the user, but these systems frequently pass quite a bit of noise with the signal. Human oversight is crucial to ensure accuracy, contextual understanding, and ethical considerations that will drive decisions of high quality and confidence.
Signal fatigue is a significant challenge in risk intelligence, where the influx of irrelevant or un-actionable signals can overwhelm teams. The Centaur Model addresses this by leveraging human judgment to filter and prioritize signals, reducing the risk of missing critical information.
As AI continues to improve, the Centaur Model remains balanced. It advocates for a collaborative approach, where humans guide AI algorithms, providing expert judgment and intuition. This ensures that risk intelligence efforts are comprehensive, accurate, and actionable, even in the face of evolving threats.
As we navigate the poly- and perma-crisis era, the Centaur Model stands as a beacon of innovation in risk intelligence. By seamlessly integrating AI algorithms with human expertise, it offers a transformative approach to risk assessment, reshaping the future of risk management for the better.
Request a Risk Intel demo to see our Situational Analysis program in action.